
Intel Speeds AI Development, Deployment and Performance with New Class of AI Hardware from Cloud to Edge | Business Wire

Analog architectures for neural network acceleration based on non-volatile memory: Applied Physics Reviews: Vol 7, No 3
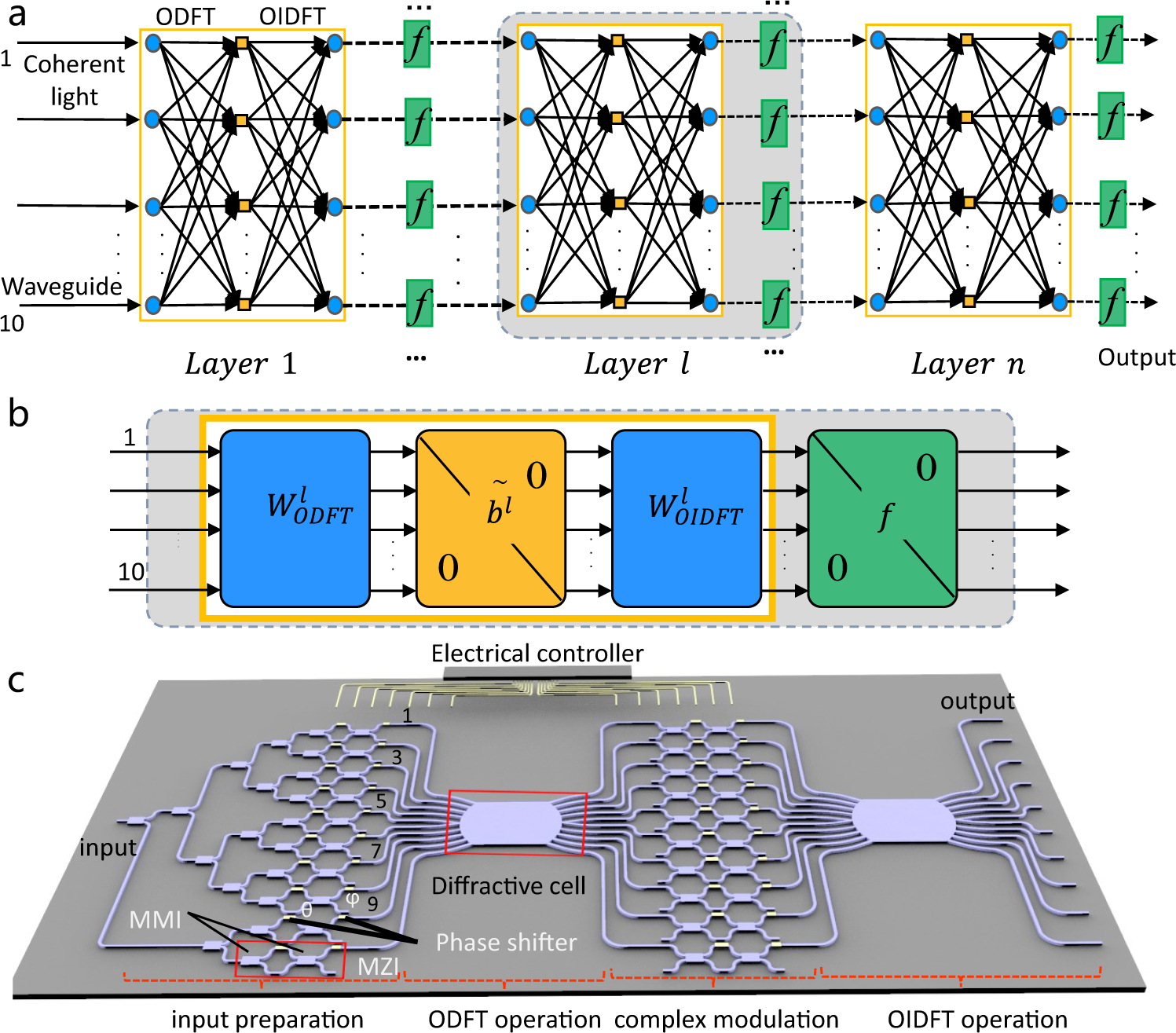
Space-efficient optical computing with an integrated chip diffractive neural network | Nature Communications
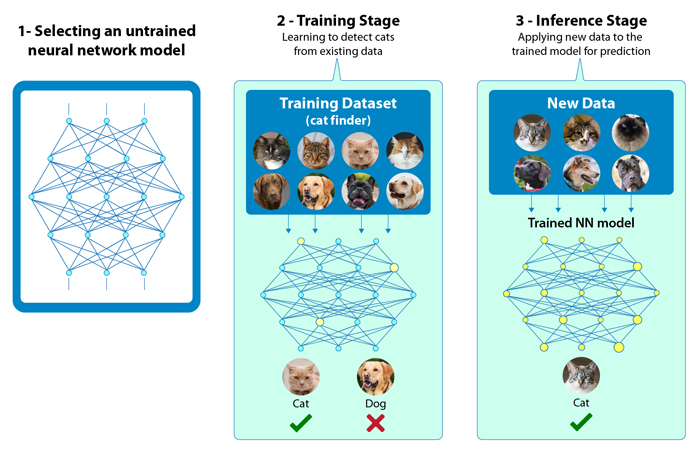
How to develop high-performance deep neural network object detection/recognition applications for FPGA-based edge devices - Blog - Company - Aldec

The New Deep Learning Memory Architectures You Should Know About — eSilicon Technical Article | ChipEstimate.com
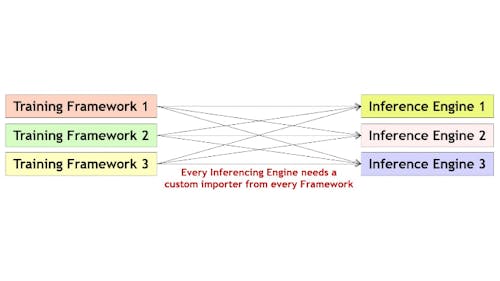